Presenter: France Rose, University and University Hospital Cologne, Germany
Audience: behavioral scientists and experimentalists using either motion capture systems or video-based tracking methods like DeepLabCut or SLEAP
Schedule: Wednesday 15th May, Room 5, 15:30 – 17:00
Description:
Pose estimation methods and motion capture technologies have opened the doors to precise and quantitative measurements of animal kinematics. However, these methods are not perfect and contain missing data which might render large portions of the recorded data unusable. In this tutorial, we will demonstrate our method: Deep Imputation for Skeleton data (DISK). DISK learns correlation between keypoints and repeated dynamics to impute missing data, based on deep learning algorithms. We developed an unsupervised training scheme, which does not rely on manual annotations. Through several testing scenarios, we have proven the usability and performance of our imputation method in six different animal skeletons, including a multi-animal set-up. With an optional estimated imputation score, DISK allows behavioral scientists to remove data with potentially high imputation error. In this tutorial, we will use a practical example to present the steps of DISK usage and show how to apply it on novel datasets. DISK as a stand-alone imputation package is designed to be used independently of the used tracking method (marker-based or markerless) and the downstream analysis, and therefore can have a wide range of uses in the behavioral science community.
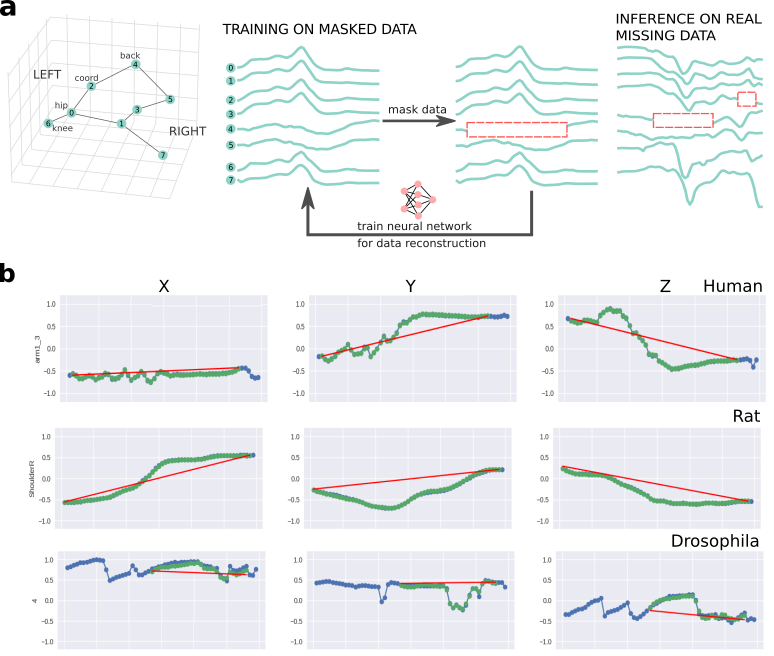