Organiser: Mona Giersberg and Bas Rodenburg (University of Utrecht, The Netherlands)
Schedule: Wednesday 15th May 10:00 – 15:00 Meeting Room 4
10:00 – 10:20 Mona Giersberg – Transdisciplinary Initiatives to Collaborate on the Responsible Use of AI for Animal Welfare
Artificial Intelligence-based methods have the potential to expand human capacities to measure and monitor animal welfare indicators more accurately and continuously. However, developing or adapting these methods requires the expertise of relatively unrelated academic fields, such as veterinary medicine and computing sciences. Therefore, collaborative structures are needed that offer perspectives beyond individual research projects. One example of such initiatives is the AI & Animal Welfare Lab at Utrecht University, which we present in this paper.
10:20 – 10:40 Marlou van der Sluis – Using Multi-Directional Computer Vision for Automated Leg Health Scoring in Broilers
Impaired walking ability is commonly seen in broilers, but there is potential to breed for improved leg health. However, walking ability in broilers is commonly assessed manually, which is time-consuming and subjective. In this study, we implement automated pose estimation and tracking by detection to assess walking characteristics in broilers from video recordings. The results of this study can aid in the development of automated broiler gait scoring approaches.
BREAK
11:10 – 11:30 Arjan van Putten – Identifying and tracking group housed hens using ArUco marker backpacks
Computer readable markers provide a method to measure individual laying hen positions in groups. We tracked and annotated videos of 2 laying hen hybrids in 20 groups of 10 hens, to examine the use of ArUco markers for identification and measurements of distance moved and average distance to other birds. Missing data due to the complex environment and challenges in the backpack design need to be overcome, but these markers show potential as standalone technology.
11:30 – 11:50 Oleksiy Guzhva – AI in rose-coloured glasses: how close to an individual animal can (should) we come?
In the diverse world of animal behaviour studies, understanding social interactions plays a crucial role in the conceptual framework of the survival and evolution of species. Recent advancements in object tracking and automated behavioural analysis, powered by new generations of GPUs and semi-automated CNN frameworks, represent significant strides in the field. This work aims to engage scientists from different backgrounds in a comparative discussion about finding the optimal sample size for automated video analysis methods.
11:50 – 12:10 Saif Agha – AI-PigNet: Insights into the social interaction of pigs through automated data and social network analysis
The aim was to explore the feasibility to use data generated from on-farm AI monitoring systems to construct social networks that describe the social dynamics of growing pigs. Results show that automated position and activity data exhibited a matching accuracy of over 97% with human observations. Social Network Analysis (SNA) of the automated data provide valuable insights into social structures and informative behavioural phenotypes. These can be employed for improving animal performance, welfare, and health.
12:10 – 12:30 Emma Baxter – Challenges and opportunities of working with PLF technology and AI researchers: the applied ethologist’s experience
As applied ethologists we are increasingly involved in projects where various aspects of AI are being developed/used as tools to identify different animal behaviours, affective states and monitor health and welfare. Projects often involve multiple stakeholders, with varied backgrounds, expertise and objectives. Such collaborations are commonplace in animal welfare science which is, innately, a multidisciplinary field. Adopting AI into this field presents exciting opportunities, but comes with different challenges, which we discuss here.
LUNCH
14:00 – 14:20 Marwa Mahmoud – Towards early prediction of disease in farm animals: Vision-AI for automatic analysis of face, gait and motion for sheep behavior understanding
Lameness is a critical indicator of sheep health conditions and is a serious animal welfare concern. Traditional methods of assessing lameness and general pain in animals are slow and costly. An automated pain estiamtoion method and lameness detection system for sheep is presented leveraging computer vision and deep learning technologies, looking at automatic analsysis of facial expressions, gait and motion behaviours.
14:20 – 14:40 Lucy Asher – Can Artificial Intelligence Contribute to our Conceptual Understanding of Animal Welfare?
Lameness is a critical indicator of sheep health conditions and is a serious animal welfare concern. Traditional methods of assessing lameness and general pain in animals are slow and costly. An automated pain estiamtoion method and lameness detection system for sheep is presented leveraging computer vision and deep learning technologies, looking at automatic analsysis of facial expressions, gait and motion behaviours.
14:40 – 15:00 Albert Ali Salah – Automatic pain estimation in equines and canines
Computer based automation creates new opportunities and challenges for animal wellbeing. While innovative applications are being implemented to assist pet owners, veterinarians, and for monitoring of animals, we also see challenges similar to those in automatic human behavior analysis, where automation raises new ethical issues and risks. We present here the problem of automatic pain estimation of equines and canines using computer vision and machine learning, and discuss the technical challenges of both.
Description:
We keep animals in various contexts: cats as companions, cows on a farm, sport horses or service dogs. As a society, we attach increasing importance to the welfare of these animals. In recent years, there has also been a paradigm shift within animal welfare science: from an emphasis on preventing welfare problems, such as pain and injury to promoting positive experiences and states in animals. Animal behaviour is a valuable indicator to assess animal welfare. In practice, however, it is often not feasible to monitor animal behaviour on a large scale and over a long period of time by human observers. This is where the added value of AI lies. With technologies such as computer vision, we can expand human capacities and monitor animal behaviour continuously and more accurately. This allows us to detect and respond earlier to behaviours that indicate a negative or positive state in animals. For this, it is important to bring together knowledge from different disciplines (e.g. veterinary science, biology, computer science), combine it and implement it in practical applications to improve the lives of animals.
This symposium brings together experts from different areas of animal behaviour science, AI and data science. We will discuss presentations on various applications of AI for measuring animal behaviour and monitoring animal welfare. These applications should put the animal at the centre and focus on how it feels and perceives its environment. The aim is to learn from each other and to explore possible collaborations to adapt successful AI tools from one field to other fields of animal behaviour science. Together, we will work to make full use of the potential of AI to improve the lives of animals in practice.
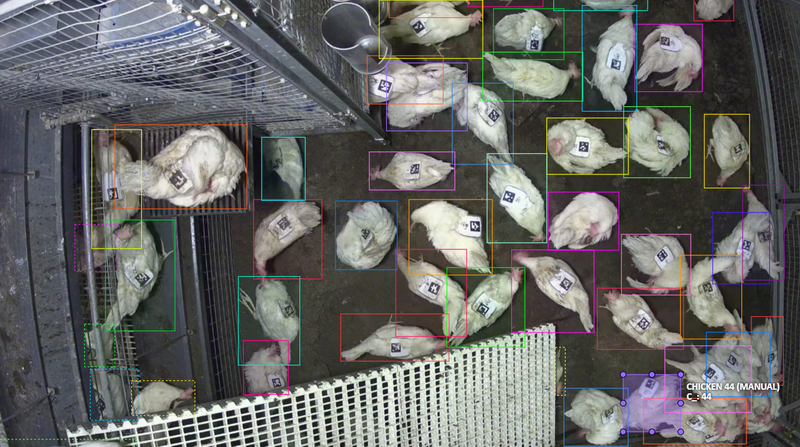